Facilitating Language Technologies for Crisis Preparedness and Response
funded by the NSF (2023-)
Project Description
Language technologies are promising and could have strong impact during disaster responses. they can help to triage text messages in a disaster to determine what aid to provide. Language technologies can translate vast amounts of data related to an ongoing pandemic. Responders can use these technologies to converse with victims during disaster responses. However, advances in language technologies to date are limited. They focus on a few dozen of the more than 6500 languages spoken or signed in the world today. Current language technologies neglect millions of people. This especially impacts those who are most at risk for experiencing disasters. This project provides an infrastructure for language technology advancements for crisis response. The results will be useful for everyone, no matter the language they speak.
This project builds datasets of crisis communications using dedicated data collections and social media harvesting. These datasets will be applicable to curated crisis scenarios. They will use common language scenarios necessary to communicate with vulnerable populations. This approach helps people for whom language technologies are not typically developed. The project will bring together researchers from different disciplines. These include language technology researchers, experts in disaster relief, linguistics, and human-computer interaction. The project will target representatives from the local speech communities to take part. To coordinate this effort, the project will organize yearly workshops and shared tasks with the communities.
This is a collaborative project between George Mason University and the University of Washington.
Participants
Faculty
Students
![]() | |
Belu Ticona, PhD CS |
Publications
- On working with epidemiological data: (Harrod* et al., 2024)
- On unlearning climate misinformation: (Fore et al., 2024)
- On using LLMs for clinical risk prediction: (Acharya et al., 2024)
Acknowledgements
This project is supported by NSF grants CNS-2234895 and CNS-2346334 under the CIRC (previously CCRI) program.
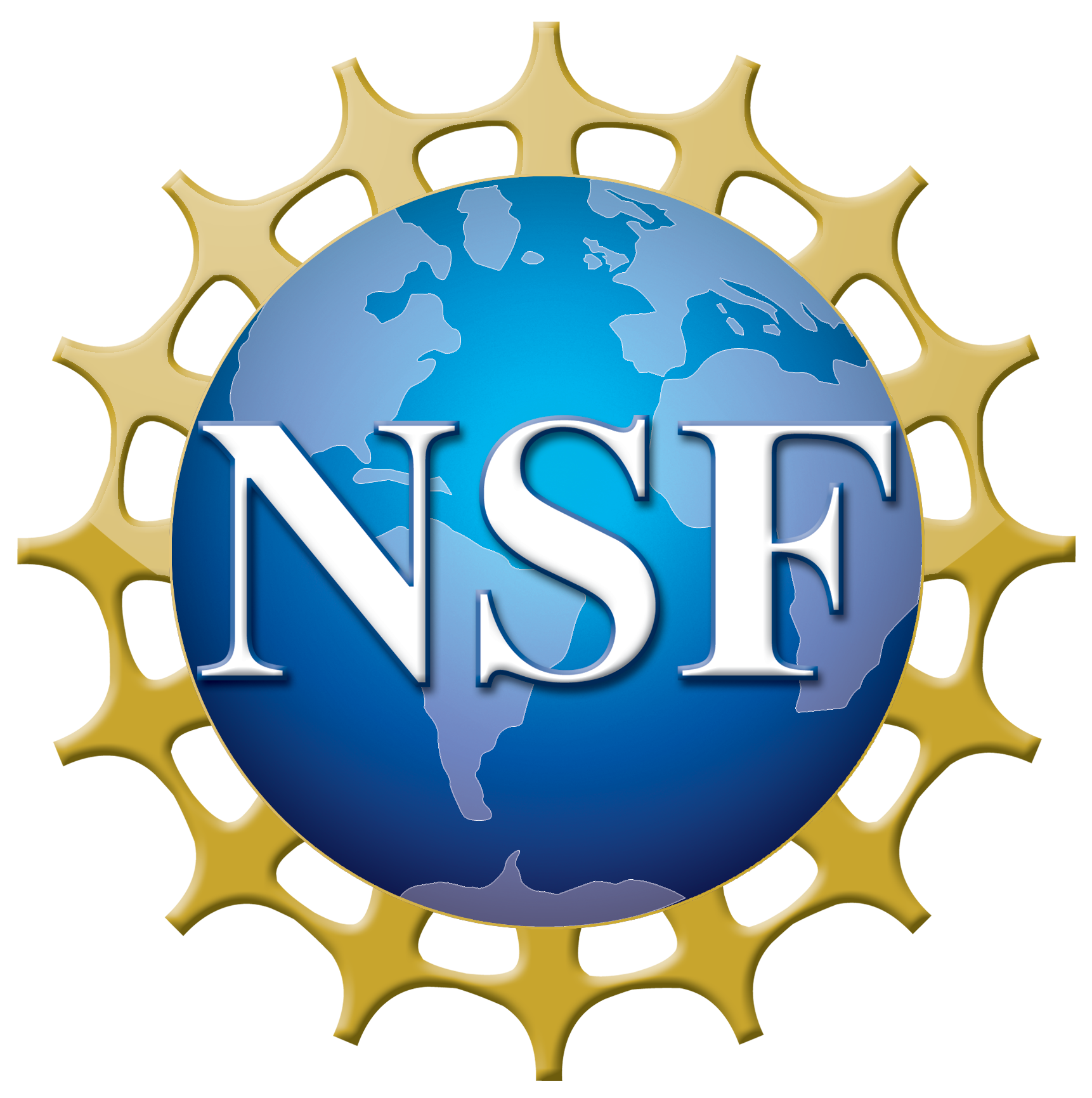